Brick-and-mortar retail cannot function efficiently without accurate logistics. From produce and groceries to consumer goods, staffing, and market trends—the list of data-driven necessities is long and extensive. The more precise your numbers, the better your outcomes.
Accurate data helps retailers navigate the constant pressure of adapting to shifting customer expectations, competitive markets, and evolving trends. Integrating retail data insights is not just an advantage but a necessity in today’s dynamic landscape. It enables businesses to predict demand, optimize inventory, and enhance customer engagement, ultimately driving operational success.
In this article, we will explore the significance of retail data insights, the different types of retail analytics, how businesses can extract actionable insights, and the real-world applications of data-driven strategies. We will also discuss the challenges retailers face in adopting analytics and how they can overcome these barriers to stay ahead in an increasingly data-driven industry.
What is Retail Data?
Not too long ago, retailers relied on intuition and experience to make decisions—guessing what customers wanted, stocking shelves based on past trends, and hoping sales would follow. But as markets became more competitive and consumer preferences evolved, guesswork was no longer enough. Retailers needed hard facts to stay ahead, and that’s where retail data emerged as a game-changer.
Retail data refers to the wealth of information collected from various touchpoints within a retail business. Every purchase made, every item stocked, and every marketing campaign launched generates data. This information provides a clear picture of store operations, customer behavior, and supply chain performance, helping retailers make informed decisions rather than relying on instinct.
At the heart of retail data are three critical components:
- Transaction Histories: Every purchase leaves behind a digital footprint. Analyzing these records helps retailers spot trends, identify bestsellers, and anticipate future demand. Understanding when and what customers buy enables better pricing, promotions, and stock planning.
- Customer Demographics: Who is shopping, and what are they looking for? Age, location, preferences, and shopping behaviors shape personalized experiences. Data-driven insights allow retailers to tailor marketing campaigns, improve product placement, and enhance customer engagement.
- Supply Chain Interactions: A smooth supply chain is the backbone of a successful retail business. Tracking supplier performance, delivery timelines, and inventory movement ensures shelves are stocked efficiently, reducing waste and avoiding stockouts. Data-driven logistics streamline operations, making retail businesses more agile and responsive.
By analyzing these elements together, retailers can transition from reactive decision-making to proactive strategies.
Importance of Retail Data Insights
Retail data insights refer to the analysis of customer behavior, sales trends, and operational data to help businesses make informed decisions.
For brick-and-mortar stores, success depends on more than just foot traffic—it requires smart, data-driven strategies. Using retail data insights allows businesses to optimize operations, enhance customer experiences, and stay competitive in a constantly evolving market. With real-time analytics, retailers can transform data into actionable strategies that improve efficiency and drive growth.
Why Retail Data Insights Matter for Brick-and-Mortar Stores:
- Boosts Business Efficiency: Identifies inefficiencies, optimizes inventory management, and streamlines supply chain operations.
- Reduces Operational Costs: Prevents overstocking, minimizes waste, and improves resource allocation.
- Enhances Customer Satisfaction: Enables personalized shopping experiences, tailored recommendations, and targeted promotions.
- Improves Demand Forecasting: Predicts sales trends, ensuring the right products are available at the right time.
- Optimizes Workforce Management: Helps allocate staff based on customer footfall patterns, reducing wait times and improving service.
- Strengthens Competitive Advantage: Provides insights into market trends, pricing strategies, and consumer preferences.
Also read: Advanced Retail Shelf Analytics for CPG Brands.
With a solid understanding of the importance of retail data insights, let’s now explore the different types of retail analytics that help businesses make data-backed decisions.
Types of Retail Analytics
Retail analytics can be categorized into four types, each serving a unique purpose in extracting actionable insights. These are as follows:
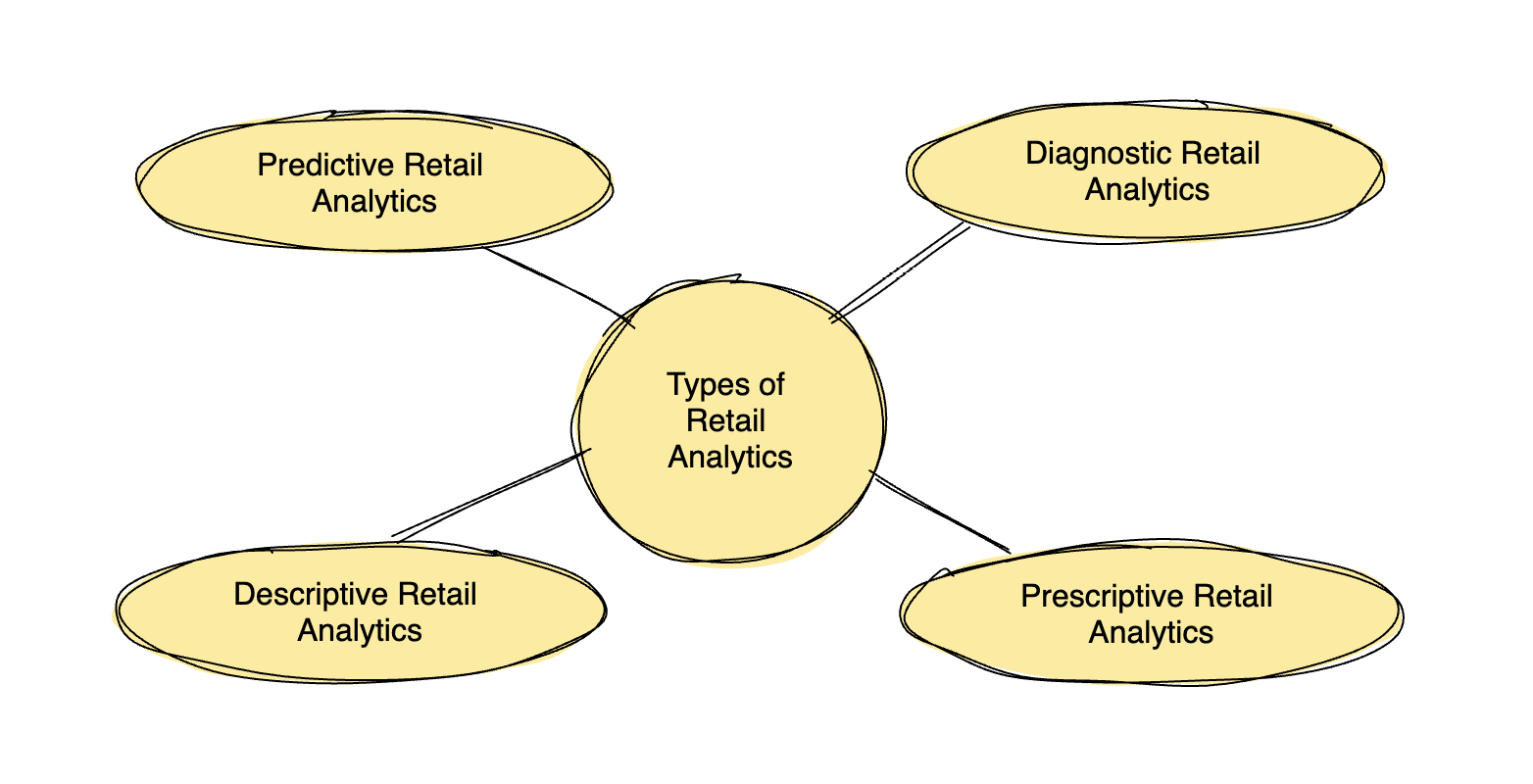
1. Descriptive Retail Analytics
Answers: “What happened?” in the past
This analytics type focuses on organizing and summarizing historical data to provide a clear snapshot of past performance. Automation tools consolidate information from multiple sources, allowing retailers to analyze sales trends, customer preferences, and inventory turnover. For example, a store can use past sales data to identify its best-performing products and peak shopping hours.
2. Diagnostic Retail Analytics
Answers: “Why did it happen?”
When sales dip, or a product underperforms, retailers need to understand why. Diagnostic analytics uncovers the root causes of such trends by using advanced statistical techniques and AI-powered tools. For instance, if a product isn’t selling well in a particular region, diagnostic analytics might reveal issues like pricing mismatches, ineffective marketing, or competitor influence.
3. Predictive Retail Analytics
Answers: “What is likely to happen?”
Looking ahead is critical for retail success, and predictive analytics helps businesses do just that. By making use of algorithms and historical data, retailers can forecast customer demand, seasonal sales fluctuations, and market trends. For example, supermarkets can anticipate increased demand for certain products before the holiday season, ensuring they stock up in advance.
4. Prescriptive Retail Analytics
Answers: “What should we do?”
Prescriptive analytics goes beyond predicting trends—it suggests the best course of action to achieve optimal results. By using simulations and machine learning, prescriptive analytics helps retailers fine-tune pricing strategies, allocate inventory efficiently, and create targeted promotional campaigns. For instance, a retailer can determine the most effective discount percentage to maximize sales while maintaining profit margins.
Also read: Realtime Retail Analytics for Improved Retail Execution
With a clear understanding of these analytics types, the next step involves extracting actionable insights through a structured data management process.
Extracting Actionable Insights from Retail Data
Transforming raw data into actionable insights is the key to driving smarter decisions and better outcomes in retail. However, to unlock the full potential of data, retailers must follow a systematic approach that involves data collection, cleansing, analysis, and visualization. These steps ensure that the insights are both accurate and relevant for improving retail performance.
Steps to Extract Actionable Insights:
- Start with Data Collection and Integration: Begin by gathering comprehensive data from various sources, including point-of-sale (POS) systems, customer transactions, inventory records, and external sources like supplier data or market trends. Ensure that this data is integrated across all systems to create a unified view of retail operations. A seamless integration allows for better decision-making by consolidating insights from different touchpoints.
- Clean and Prepare the Data: Raw data is often messy and may contain errors or inconsistencies that can distort analysis. To ensure the data is reliable, cleanse and organize it by removing duplicates, correcting errors, and standardizing formats. For example, standardizing product categories and fixing inconsistencies in customer details ensures that your data is accurate and ready for deeper analysis.
- Conduct Data Analysis: Use descriptive, predictive, and prescriptive analytics to identify meaningful patterns and trends within the data. For example, analyzing past customer purchase history can reveal peak shopping times, helping you plan staffing and inventory needs more effectively. Look for trends in customer behavior, sales fluctuations, and inventory turnover that can guide operational decisions.
- Visualize and Report the Insights: Transform complex data into clear, actionable insights by using visualization tools like Power BI or Tableau. Create real-time dashboards that display key metrics in an easy-to-understand format, enabling quick access to crucial information. These visual reports not only highlight areas needing attention but also support fast, informed decision-making at all levels of the business.
Once actionable insights are extracted, they can be applied to various retail operations to achieve measurable results.
Applications of Retail Data Analytics
Once actionable insights are extracted, retailers can apply them across various areas to enhance efficiency, improve customer experiences, and drive profitability. By using data-driven strategies, businesses can make informed decisions that optimize performance and reduce inefficiencies. Here are some key applications of retail data analytics:
- Business Intelligence: Tools like Power BI and Tableau help retailers monitor key performance indicators (KPIs) and uncover growth opportunities through advanced visualizations. Visual dashboards can, for example, pinpoint underperforming stores or products.
- Sales and Demand Forecasting: Predictive analytics allows retailers to anticipate demand, ensuring they stock the right products at the right time. Seasonal forecasting helps optimize stock levels, reduce waste, and maximize sales.
- Optimizing Supply Chain: Real-time analytics provide insights into stock levels, transportation networks, and supplier performance, enabling better decision-making to reduce delays and improve delivery efficiency. Retailers can, for instance, identify bottlenecks and streamline delivery timelines.
Armed with these applications, retailers can implement insights to revolutionize their operations and stay ahead in the competitive market.
Having explored the practical applications of retail data analytics, it's clear how these insights can drive business efficiency and profitability. However, to truly capitalize on these benefits, retailers must also address the challenges that come with implementing data-driven strategies. Let’s now take a look at the common hurdles and how overcoming them can help unlock the full potential of retail analytics.
Using Retail Data Insights for Business Growth
Retailers who harness data insights effectively can revolutionize marketing, streamline inventory management, and develop strategic plans that align with market trends. However, implementing retail analytics comes with challenges that businesses must overcome to unlock its full potential.
The table below outlines how data insights enhance business performance and the key challenges retailers face in their analytics journey.
.png)
Also read: Real-time Retail Analytics to Drive Retail Execution.
By addressing these challenges—such as upgrading outdated systems, integrating data sources, and improving collaboration—retailers can maximize the benefits of data analytics, driving long-term growth and competitive advantage.
With a solid understanding of how retail data analytics can enhance business growth, it's time to explore how advanced solutions like ParallelDots' AI-driven tools can further amplify these efforts. These tools help retailers streamline operations, optimize inventory management, and improve customer engagement—all essential components of driving long-term growth and staying ahead in a competitive market.
Transforming Retail Performance with ParallelDots' Data Analytics & Insights
ParallelDots' suite of AI-driven solutions empowers retailers to maximize the value of their data by providing real-time insights and intelligent analytics. With tools like ShelfWatch, Saarthi, and SmartGaze, ParallelDots helps retailers optimize in-store execution, improve stock management, and enhance customer engagement—ensuring that data back every decision.
Key Features that Drive Retail Transformation:
- ShelfWatch: Utilizing computer vision and AI, ShelfWatch helps retailers monitor product placement, track shelf availability, and ensure planogram compliance. This results in better stock management, reduced waste, and improved sales by maintaining optimum shelf stock levels.
- Saarthi: A comprehensive solution that focuses on optimizing retail operations, Saarthi allows businesses to track inventory, plan and forecast demand, and manage suppliers efficiently. It integrates seamlessly into existing systems, ensuring a streamlined approach to data-driven retail management.
- SmartGaze: This tool automates gaze-coding for eye-tracking research, enabling businesses to analyze customer attention and optimize product placement and in-store marketing strategies. The result is a more engaging and personalized shopping experience that drives conversions.
With these tools, retailers can transform their operations, ensuring that their decisions are informed, timely, and impactful. ParallelDots brings together the power of AI and data analytics, helping businesses stay ahead of trends, optimize supply chains, and improve customer satisfaction.
Book a demo with us today to see how ParallelDots' AI-driven solutions can help you unlock actionable insights, streamline your operations, and drive growth. Let’s make data work for you!