The present shelf arrangement KPI assessments using your standard retail execution software, are often time-consuming and difficult to manage around work-peak. Meticulous manual input is required to guarantee that products on the shelf match with the planogram. Moreover, the lack of visibility and up-to-date data prevents consumer goods brands from tackling problems proactively. During a crucial sales period, a lack of data can lead to suboptimal decisions.
According to a study, “As many as 81% of companies reported that they’re unsatisfied with their ability to execute at retail. Another 86% said they’re not satisfied with their trade promotion efforts”.
With ShelfWatch, all these redundancies can be tackled quite easily. A powerful and hassle-free tool, ShelfWatch is capable of running on a wide spectrum of retail channels. In this blog, we walk you through all the aspects of ShelfWatch that make it stand out amongst the existing Image Recognition Software solutions in retail.
1. Real-Time, Offline Image Quality feedback
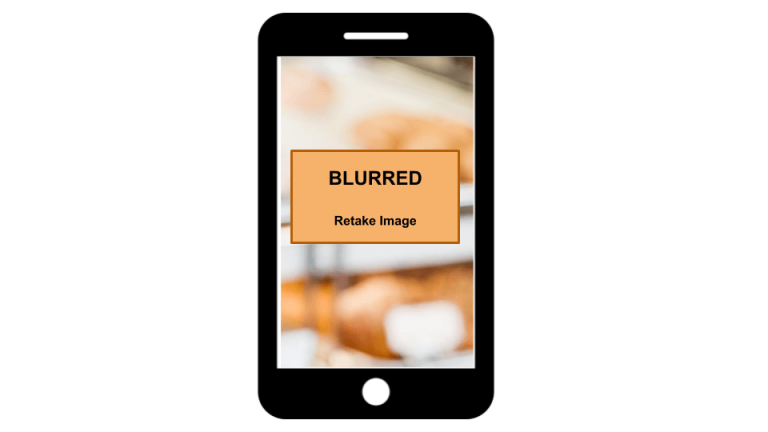
Image Quality is an important criteria to ensure the high accuracy of Image Recognition. SKU level recognition or price display compliance is only possible when the image is not blurry and free from glare. The ShelfWatch mobile app has a real-time image quality algorithm that can detect poor quality images and instruct the sales rep to retake photos. This detection works on the device and therefore, is available in offline mode.
The sales reps can easily take high-quality images even in a no-internet zone and the images are automatically uploaded whenever an internet connection is available. In our experience of working with CPG & retail brands, we found that before using ShelfWatch, 15–20% of the images collected in the field were too low quality to be analyzed by AI or in many cases, by humans as well. This often leads to unnecessary delays and incomplete analysis. Existing retail execution software put the blame on sales reps in case of blurry or glary photos, and put the onus on CPG & retail brands to train their busy reps.
An ideal retail execution software that uses image recognition, should be robust and smart to ensure high-quality photos are collected without any additional training for the reps.
2. On-Device Image Recognition (ODIN)
One of the biggest limitations of AI-enabled audit solutions is to give accurate results instantly. To deliver high accuracy, computing power required is high. However, handheld devices used by reps have limited compute resources and one has to be careful to avoid excessive battery consumption of reps’ device lest he needs to charge his device after every 2 or 3 visits. This is where ParallelDots’ ODIN solution wins. Our data science team has succeeded in optimizing our algorithm in such a manner, that ShelfWatch gives you the best of both worlds – accuracy, and speed.
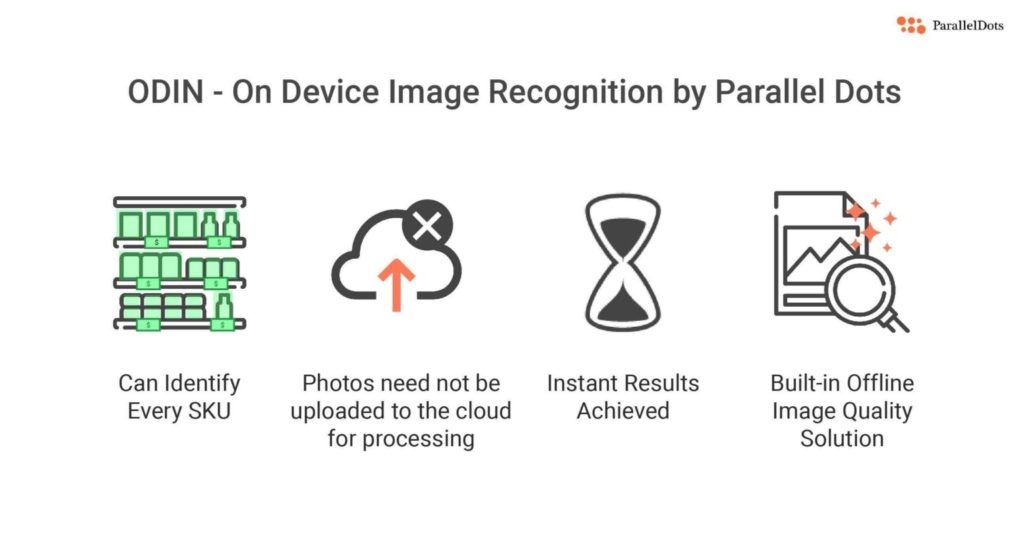
On-Device Image Recognition (ODIN) is the most cutting-edge offering from the ParallelDots stable. It allows instant reporting from shelf photos captured by the field reps by processing them on their hand-held device. ODIN is fast and works completely offline. We ran pilots with a couple of clients for the recently announced on-device recognition feature. The results are encouraging and have exceeded client expectations. The ODIN feature is a unique offering and testament to our superior image recognition platform for a retail environment. We encourage clients to use the ODIN feature for domains where low number of SKUs are involved, and they undergo infrequent changes.
3. De-duplication

Very often it happens that while collecting data, the sales reps take multiple images of the same shelf from various angles. This is a serious problem as it may lead to double counting of shelf metrics (such as share of the shelf) which in turn, affects insights. ShelfWatch masters this problem very efficiently. Its de-duplication algorithm improves data quality by detecting duplicate images and ensuring the metrics are not double-counted.
We also leveraged this algorithm to detect fraud in regular audits of retail execution for a tobacco company. The field auditors would often submit an old image to indicate that they have completed the audit. Using the de-duplication algorithm, we were able to surface such instances and reduce the possibilities of fraud in field audits. Within three months of integrating ShelfWatch, there was a 90% improvement in the data quality leading to reliable insights.
4. Integration with other retail execution software - SFA and DMS apps
While ShelfWatch provides its own app for capturing data in the field, we do understand that sales reps are already using handheld provided by the Salesforce automation vendors and will find it cumbersome to switch between multiple apps in the field.
We have integrated ShelfWatch with multiple SFA vendors and all the features of ShelfWatch such as real-time image quality checks and real-time shelf insights work in the integrated solution as well.
5. Quick Setup & Fast to train AI
Under the hood, most of the Image Recognition engine runs a neural network to detect SKUs and POS Materials in retail stores. However, neural networks, especially deep neural networks are notorious for needing a large amount of data to train them and get 90% and above accuracy.
Also, the training data needs to be manually annotated before it can be fed to the neural network. An example of an annotated image is shown below.
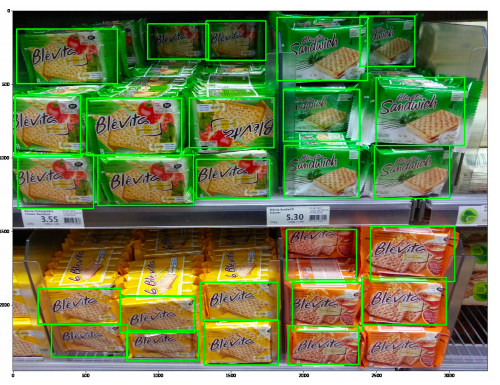
However, a large manufacturer will have 200–300 SKUs across multiple categories of their own brands and another 100–200 SKUs that they may want to track for their competitors. Generating a manually annotated dataset that covers 300–500 SKUs is a tedious and very expensive task.
Most Image Recognition vendors will take 90–120 days setup time during which they collect and manually annotate data. As you can imagine, this is an expensive and time-consuming process and does not scale well for new product launches or during peak promotions time.
Setting up Shelfwatch is a simple, two-step straightforward process. First, you need to share only one image of the SKUs that you want to track. And second, ask your field reps to take images of the retail outlet’s shelves using our mobile application. ShelfWatch’s algorithm is trained in such a manner that it automatically analyzes the images to give out a competitive analysis like share-of-shelf, and planogram compliance.
6. Cost-Effective
ShelfWatch has been made with state-of-the-art technology to give out optimum results without having to spend a lot of money. With our superior technology, we support low operational costs because of the fewer resources required in setting up ShelfWatch. Our algorithm controls the data quality at the collection level to bring out the standard, objective analysis.
7. WhatsApp Alerts -
The real value from ShelfWatch is derived when all instances of below par retail execution are instantly highlighted to the right stakeholders. We send automatic alerts via WhatsApp/email to field team leaders for quick interventions. This new offering makes ShelfWatch insights more actionable - leading to a robust feedback mechanism between the retailer, field rep and the CPG HQ.
ISO 27001:2013 Certification -
It is with immense pleasure that we announce that we are now ISO 27001:2013 certified. To achieve the certification, ParallelDots’ security compliance was validated by an independent audit firm after demonstrating an ongoing and systematic approach to managing and protecting company and customer data. This certificate is a testament to our commitment to data privacy and security.

Found this blog useful? Read this blog to know more about how ParallelDots products provide effective solutions to traditional retail execution methods to improve brand presence and visibility.
Want to see how your own brand is performing on the shelves? Click here to schedule a free demo.