Automated retail audits using Image Recognition have gained popularity in the recent years with many CPG manufacturers trialing the solution, or in advanced stages of implementing it globally. However, as per the POI report, cost and speed are main concerns, preventing the widespread adoption of this game-changer solution
Image Recognition for tracking retail execution is becoming popular because of the time savings and high accuracy it can deliver as compared to manual store checks. As per Gartner report, Image recognition technology can increase sales force productivity, improve shelf condition insights and help drive incremental sales.
Despite all the proven benefits of Image Recognition technology, practical issues such as high cost of implementation and slow turnaround times have kept the adoption of this solution low. We, at ParallelDots, have been hard at work trying to tackle these issues by launching our on-device image recognition solution, ODIN. With ODIN, all the images captured by reps will be processed on their hand-held device, thus doing away with the need of using an active internet connection and quality check processes to generate KPI reports. In this blogpost, we will discuss our approach on ODIN and why this could be game-changing for CPG companies of all sizes, who want to implement their perfect store programmes.
Why On-Device Image Recognition is game changing for Automated Retail Audits
Current state-of-the-art image recognition algorithms need powerful servers such as GPUs to perform efficiently. This type of compute power can be made available through modern-day cloud computing infrastructure. However, this means that as field reps are taking photos in the store, these photos need to be uploaded to the cloud servers before shelf KPIs can be calculated from these photos. This process works well in stores with Wi-Fi connection or good 4G internet connectivity.
However, internet connectivity may not be good in many areas or with underground stores. For such stores, getting a KPI report is not possible while the rep is still in the store. For such cases, on-device image recognition could work very well to ensure that reps get feedback on the photos they are taking, without requiring them to go online.
Also, Image Recognition technology works well on high-quality images. Which means, it may take a while for the images to get uploaded, even in areas that offer decent network availability. This may lead to scenarios where field reps have to wait for additional time before their images are uploaded, processed in cloud server and then, the results are sent back to the rep. On-device recognition eliminates this issue and produces the result instantly. Field reps get to have insights in seconds, rather than waiting for 5-10 minutes. This makes the output more actionable, and no time is spent in waiting for AI analysis.
Challenges Involved-

For conducting automated retail audits using image recognition to work effectively, good quality images are required. Even small changes in the image quality can lead to dip in accuracy when performing image recognition. This is paramount to the accuracy of the computer vision model that is running on the device.
Also, getting the right amount of high-quality training data for the purpose of image recognition can be challenging. Almost none of the CPG manufacturers have a readily available labelled database of store images. Thus, one of the biggest hurdles to getting started with on-device image recognition is the lead time and costs associated with creating such a database.
Moreover, new products are launched or product packaging is changed - therefore constant training and retraining of AI is performed to keep it updated. Add to the fact that the high amount of data for new product launches would take some time to accumulate, before the AI can even be trained on the same.
Some facts to consider before opting for on-device image recognition -
There is always a trade-off between accuracy and speed of insights and therefore, an ideal solution will find the most optimum value to make the solution practical. Therefore, CPG executives will have to assess what will be the impact of a lower accuracy or slower insights before opting for on-device image recognition.
It's important to note that here we are referring to small differences in accuracy and speed as we acknowledge that an ideal solution will be accurate and very fast. A CPG manufacturer may be able to deploy a 91% SKU level accurate model on-device with lower setup time and costs, than what it could take to deploy a 98% accurate model. However, if high accuracy is critical for them (due to retailer incentives), they may opt for online image recognition which allows for a quality check process to ensure higher accuracy. However, this means that reps will have to wait for the images to get uploaded, processed, quality checked and then, wait for the report to be downloaded on their device before they can access the KPIs.
For practical purposes a 91% solution may also work. A 91% accurate solution would mean that out of say, 50 unique SKUs available on the shelf, AI may not pick ~4 SKUs correctly. Given the amount of time that the field reps may save courtesy on-device recognition, it may be a better compromise than letting them wait for the reports generated in the online mode (even if it may be 98% accurate). They can simply ignore the incorrect predictions made by the AI and take action on the correct ones.
Using this solution is similar to asking Siri to play a song, most of the time she will correctly understand the song we asked her to play but on a few occasions, she may not understand our request and play a different song. In my own test with Siri, I have found it to be 80% accurate when it comes to playing songs from my voice command as out of ten requests, she could not fulfill my two requests. Yet, a compromise I’m more than willing to take since opening an app, browsing or searching for a song is more cumbersome (100% accurate solution) than asking Siri to play it.
ODIN by ParallelDots : On-Device Image Recognition for Automated Retail Audits
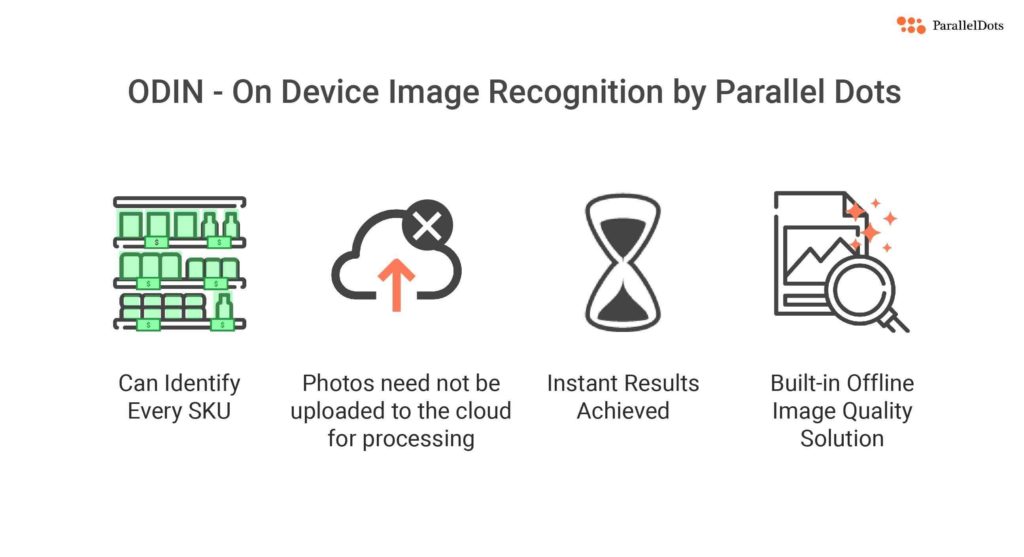
One of the biggest limitations of AI-enabled audit solutions is to give accurate results instantly. To deliver high accuracy, computing power required is high. However, handheld devices used by reps have limited compute resources and one has to be careful to avoid excessive battery consumption of reps’ device lest he needs to charge his device after every 2 or 3 visits. This is where ParallelDots’ ODIN solution wins. Our data science team has succeeded in optimizing our algorithm in such a manner, that ShelfWatch gives you the best of both worlds - accuracy, and speed.
With ODIN, our solution can identify every SKU in the photo and its location without requiring the photos to be uploaded to the cloud for processing. This means that reps can instantly see the missing SKUs as per the MSL list and identify the wrongly placed SKUs (such as putting premium brands at the bottom shelf). ODIN also has a completely offline image quality grading solution built into it which prompts the rep to retake photos if the photos are not of the optimum quality to do photo recognition.
When it comes to on-device image recognition, we recommend our clients to deploy it for a limited number of SKUs and KPIs. Also, since quality checks are not possible with on-device processing, it is important to train a very accurate model before the start of the project to ensure AI has seen enough samples of every SKU in different environments and under different orientations. Therefore, we recommend our client a longer setup period to collect high-quality data and then train a model on it. Once deployed, ODIN still needs human feedback, and we ask reps to provide feedback on the model’s output such that AI can learn from those feedbacks and become better.
How to prepare for on-device image recognition -
On-device image recognition brings with it immense scope. For implementing it successfully, certain preparations are required. Our recommendation is to firstly start with the online mode and let the AI be trained on a variety of SKU images before then moving to the on-device mode. The CPG can first deploy their top retail visibility KPIs in the on-device mode.
Moreover, strategic insights like competitive information and price display recognition can be tracked in online mode since this may not necessitate quick, remedial actions.
The CPG should also ensure that their field reps are well-trained when it comes to the guidelines of capturing ideal images. This would be helpful in producing highly accurate SKU recognition reports before switching to the on-device mode.
On-device image recognition is one of the key features that would help CPG manufacturers to keep their eyes on even their remote stores and improving the retail execution for them. The impact of reps being able to action the instant reports can then lead to improved customer satisfaction, leading to improved brand health and better sales. In the post-COVID era, customers will not give a second chance to those brands who have fluctuating availability on the shelves as they will opt for an alternate product or move to e-commerce channels.
Liked the blog? Check out our other blogs to see how image recognition technology can help brands improve their execution strategies in retail.
Want to see how your own brand is performing on the shelves? Click here to schedule a free demo for ShelfWatch.